Computational and Data Science Seminar September 15, 2023 with Ph.D. students Momina Liaqat and Richard Hoehn
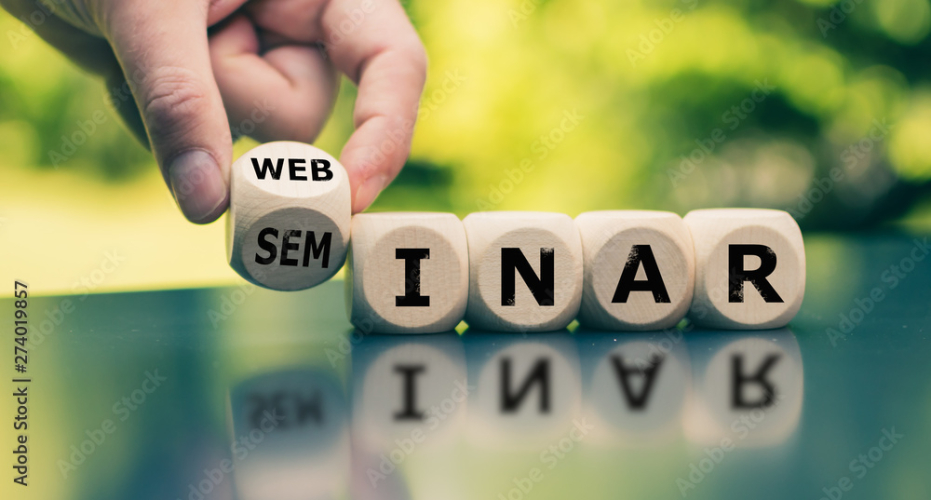
Channel boosting-based object detection and segmentation for cancer analysis in histopathological images.
Momina Liaqat
Cancer is one of the most frequent and lethal diseases on the planet. Lymphocytes are thought to be a cancer sign because they concentrate near the location of tumors as a result of the immune system's reaction. The identification and then quantification of lymphocytes are crucial in evaluating the course of cancer and the efficiency of treatment. On the other hand, a detection system based on machine learning techniques faces a variety of difficulties, including unavailability of annotations lymphocytes aren't always represented well, and the presence of irregular stains and artifacts that provide the false appearance of lymphocytes on tissues. All these contribute to making lymphocyte detection a difficult task. Also, manual detection and identification takes a lot of time for the pathologists to carefully examine each whole slide image. Manual tasks can also be prone to errors because of human subjectivity. These shortcomings raise the need for a digitally automated system that can lessen the burden on pathologists and perform the detection task with good accuracy. In this study, the goal is to create an automated lymphocyte identification system in histopathology that can overcome all the problems we face with conventional detection techniques.
To achieve automated lymphocyte detection, the Channel Boosting idea is exploited to enhance the feature space by using different feature extractors as auxiliary learners. The dataset used in this study is taken from Grand Challenge and the dataset has a total of 20,000 instances. Because of the good performance of the proposed model which was evaluated based on the F-score, this model may assist pathologists in automated detection of lymphocytes.
Improving Emotion Detection Through Translation of Text to ML Models Trained in Different Languages
Richard Hoehn
This research paper investigates enhancing Emotion Detection (ED) by translating extended text data into various Machine Learning (ML) models trained in distinct languages. We focused on English and German text data to enhance prediction accuracy, aiming to overcome challenges arising from limited labeled datasets and language fragmentation in ED research.
Expanding an original English dataset with translated German data increases the training data's volume, potentially improving prediction rates in ED applications. Additionally, translating English to German to extend the German dataset and accessing in real-time ML models trained in both could further improve prediction rates.
For presentation purposes, datasets in both English and German were collected, parsed, cleaned, and translated. Multiple ML models trained in English and German where built, and made accessible via an API for predictions in either language including real-time translation using a GET method.
The research findings suggest that the extension of datasets through translation has not yielded improvements in predictive accuracy for both English and German languages. Modest enhancements could potentially be achieved by concurrently accessing English and German models through real-time translation via the RESTful API; however, the benefits may not fully justify the efforts.
We posit that the prevalence of numerous classes in this multi-class classification model has contributed to instances of overfitting across several labels/classes. This occurrence, in turn, has led to a memorization effect rather than facilitating genuine learning within the models.
In conclusion, it becomes evident that a more substantial accumulation of data is required, or an innovative approach involving the utilization of AI to generate analogous data must be employed to comprehensively address this research question.
Watch the seminar here.